From Reactive to Proactive: AI's Role in Predictive Network Maintenance
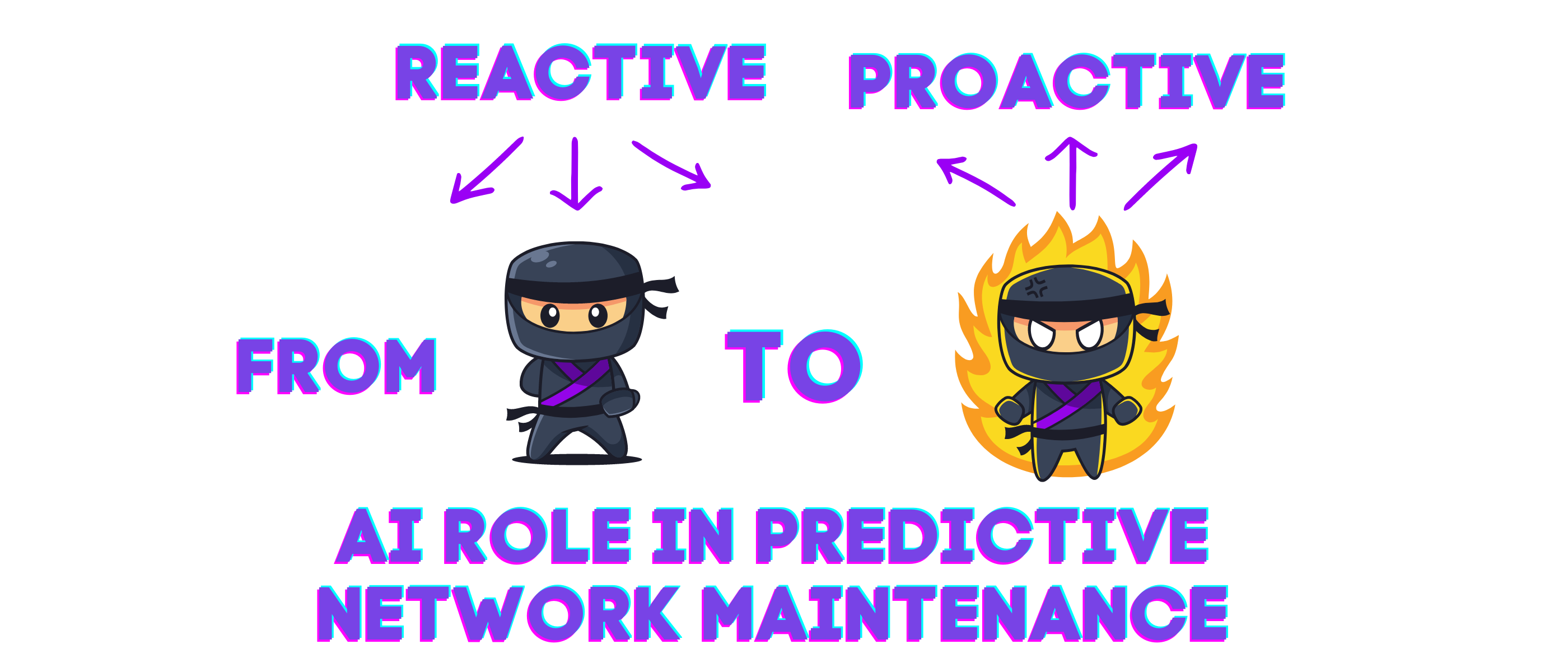
In this comprehensive article, we will be talking about Artificial Intelligence's transformative role in shifting the network maintenance paradigm from a reactive to a proactive approach.
The article covers a range of topics:
- Introduction to Reactive vs. Proactive Maintenance: An overview of traditional reactive maintenance practices and the emerging trend of proactive maintenance enabled by AI.
- The Technologies Behind Proactive Network Maintenance: A deep dive into the AI technologies, including machine learning and neural networks, that are the driving forces behind proactive maintenance strategies.
- Practical Applications of AI in Predictive Network Maintenance: How AI technologies like machine learning and neural networks revolutionize real-time monitoring and predictive analytics in network maintenance.
- Challenges and Ethical Considerations: A look at the complexities of implementing AI, from data privacy concerns to ethical dilemmas and cost factors.
- The Future of AI in Network Maintenance: Insights into upcoming advancements and trends, including quantum computing and ethical AI frameworks.
- Final Conclusion: A wrap-up of the article emphasizes AI's transformative impact on the maintenance of networking devices, the challenges to overcome, and the exciting future that lies ahead.
By the end of this article, you'll gain a comprehensive understanding of how AI is not just an auxiliary tool but a pivotal element in modernizing network maintenance strategies. Whether you're a network engineer, a tech enthusiast, or a business leader, this article offers valuable insights into the future of network maintenance in the tech industry.
Introduction: The Paradigm Shift in Network Maintenance
In today's fast-paced digital world, network maintenance has evolved from a reactive approach to a more proactive model, thanks to advancements in artificial intelligence (AI). Gone are the days when network teams would scramble to fix issues after they occur. With the advent of AI technologies like machine learning and predictive analytics, we as humans can now anticipate potential issues in our network and address them before they develop and escalate into high-risk situations, affecting customers and leading to costly downtime.
The Limitations of Reactive Network Maintenance
Traditionally, reactive maintenance has been the go-to approach for most organizations regarding network maintenance. In this model, network equipment is repaired or replaced only after failure. While this might seem cost-effective in the short term, the long-term maintenance costs can be staggering. Unexpected failures can lead to downtime, lost revenue, customer disappointment, and even reputational damage. Moreover, reactive maintenance often involves computationally expensive processes and analyzing large amounts of data, making it less efficient.
The Rise of AI in Proactive Network Maintenance
Artificial intelligence, particularly machine learning and predictive analytics is revolutionizing how we approach network maintenance. These technologies harness the power of data to predict equipment failures and schedule timely interventions. By analyzing patterns and trends in data sources, AI tools can identify the major ones that indicate an impending failure. For example, natural language processing algorithms can look through large amounts of unstructured data like networking logs to identify anomalies or errors the human mind can't think of or identify.
Predictive Analytics: The Cornerstone of Proactive Network Maintenance
Predictive analytics tools use advanced algorithms to analyze network data and make future predictions. This form of analytics is particularly useful in identifying potential issues before they become major problems and cause downtime, leading to high costs. It employs various aspects of machine learning, including artificial neural networks and deep neural networks, to analyze data and predict outcomes. These technologies are increasingly integrated into modern AI solutions for network maintenance tasks.
The Business Value of Proactive Network Maintenance
Switching from a reactive to a proactive maintenance model offers significant business benefits. Not only does it reduce network maintenance costs, but it also improves performance, lowers support needs, and extends the lifespan of equipment. Businesses can achieve massive savings and boost their bottom line by adopting AI-driven proactive network maintenance strategies.
Moreover, using AI in network maintenance aligns well with broader business objectives, offering value in various aspects like customer satisfaction, operational efficiency, and other business areas.
Conclusion
As we delve deeper into the era of AI and machine learning, the shift from reactive to proactive maintenance is not just a trend but a necessity. Organizations that fail to adapt risk falling behind and facing the consequences of outdated maintenance practices.
The Technologies Behind Proactive Network Maintenance
Machine Learning: The Backbone of Predictive Network Maintenance
Machine learning (ML), a subset of artificial intelligence (AI), drives predictive network maintenance. It enables systems to learn from data, identify patterns, and make decisions without human intervention. Machine learning algorithms analyze vast amounts of data from various sources, such as sensors and logs, to predict equipment failures and recommend preventive actions. These algorithms are often based on artificial neural networks, including deep neural networks, which mimic the human brain's ability to process information.
Artificial Neural Networks and Deep Learning
Artificial neural networks are computational models inspired by the human brain's neural networks. They consist of interconnected artificial neurons that process information in layers. Deep learning, a more advanced form of artificial neural networks, involves building multiple layers of these neurons, known as the "next layer," to solve problems that are computationally expensive and complex. These technologies are particularly effective in handling unstructured data, such as images and natural language processing tasks.
Natural Language Processing: Making Sense of Unstructured Data
Natural language processing (NLP), another subset of AI, plays a crucial role in predictive maintenance. It enables the system to understand and interpret human language, making it possible to analyze maintenance logs, customer feedback, and other forms of unstructured data. NLP algorithms can sift through this data to identify key factors that may lead to equipment failure, thereby reducing maintenance costs and improving system performance.
Predictive Analytics and Data Sources
Predictive analytics extends beyond mere algorithms; it's equally about the caliber and volume of data involved. A blend of real-time and historical data from diverse data sources is crucial for effective predictive maintenance in enterprise networking. These sources could range from network traffic logs and device status reports to latency metrics and bandwidth utilization. Utilizing machine learning and artificial intelligence, analytics tools sift through this data to generate actionable insights that preemptively identify potential network issues and inform maintenance activities.
The Human Element: Bridging AI and Network Maintenance Practices
While AI and machine learning offer numerous benefits, the human element cannot be overlooked. Network teams must be trained to interpret the insights generated by AI technologies and apply them in real-world scenarios. For example, training data must be carefully curated by to ensure that the machine learning models are accurate and reliable. Human intelligence is essential for fine-tuning these models and for making sense of the data that AI systems generate.
Conclusion
The technologies that power proactive maintenance are diverse and complex, ranging from machine learning and artificial neural networks to natural language processing and predictive analytics. Technologies are transforming maintenance practices, making them more efficient and cost-effective.
Practical Applications of AI in Predictive Network Maintenance
AI-Driven Network Monitoring: A Game-Changer for Network Engineers
For network engineers, the traditional approach to maintenance has been largely reactive. However, AI technologies like machine learning and neural networks are revolutionizing this space, enabling a shift to proactive network maintenance. AI-driven network monitoring tools can analyze vast amounts of data in real time, identifying potential issues before they escalate into major ones. This proactive approach not only reduces downtime but also significantly cuts maintenance costs.
Predictive Analytics for Network Optimization
Predictive analytics software powered by AI can provide invaluable insights into network performance. These analytics tools use historical and real-time data to forecast network behavior, allowing engineers to take preemptive actions. For example, if a particular server shows signs of overload, predictive analytics can alert the team to redistribute the load, thereby preventing a potential system failure.
AI in Cybersecurity: Safeguarding Network Integrity
Network security is a high-risk area that can't be ignored in today's world. AI technologies like deep learning and artificial neural networks can analyze unstructured data to identify unusual patterns or potential security threats. This artificial intelligence enables network engineers to act before a cybersecurity incident occurs, safeguarding the integrity of the network and protecting valuable data.
Automating Routine Tasks: AI as a Network Assistant
AI can also automate many of the routine tasks that network engineers handle daily. From configuring network settings to updating software, AI-driven tools can perform these tasks more efficiently, freeing up engineers to focus on more complex issues. This not only improves the performance of computers on the network but also enhances the job satisfaction and effectiveness of the human workforce.
Conclusion
Integrating AI technologies into network maintenance is proving to be a game-changer for the tech industry. By leveraging machine learning algorithms, neural networks, and predictive analytics, network engineers can transition from a reactive to a proactive approach. This shift is beneficial in reducing maintenance costs and improving network performance; it also has the potential to revolutionize how network engineers operate, making their roles more strategic and impactful.
Challenges and Ethical Considerations in Implementing AI for Predictive Network Maintenance
Data Privacy and Security: A Double-Edged Sword
While AI technologies offer advanced capabilities for predictive network maintenance, they raise concerns about data privacy and security. Network engineers must ensure that their AI tools comply with data protection regulations. This is particularly crucial as these tools often require effective access to sensitive network data.
Ethical Considerations: AI's Decision-Making Process
AI algorithms can make decisions that significantly impact network performance and security. Network engineers must be aware of the ethical implications of these decisions. For instance, if an AI system wrongly identifies a network packet as a security threat, it could lead to unnecessary disruptions in service.
The Cost Factor: ROI and Maintenance Costs
Implementing AI solutions for predictive network maintenance can be costly. Network engineers must conduct a thorough cost-benefit analysis to ensure that the investment in AI technologies will yield a positive return on investment (ROI). This involves evaluating how much the AI system can potentially reduce maintenance costs and improve network performance.
Skill Gap: The Need for Continuous Learning
The rapid advancements in AI technologies necessitate continuous learning for network engineers. As neural networks and AI tools become more complex, there is a growing need for engineers to understand not just the networking aspects but also the underlying AI algorithms. This is essential for effective implementation and troubleshooting.
Conclusion
Implementing AI for predictive network maintenance in the tech industry comes with its own set of challenges and ethical considerations. From data privacy concerns to the cost factor and the skill gap, network engineers must navigate these complexities carefully. However, the potential benefits, such as reduced downtime and maintenance costs, make it a worthwhile endeavor for those willing to tackle these challenges head-on.
The Future of AI in Predictive Network Maintenance: Trends and Advancements
The Rise of AI-Driven Network Solutions
As we look to the future, AI-driven solutions are set to dominate the landscape of predictive network maintenance. Advanced machine learning algorithms and neural networks are becoming increasingly sophisticated, offering unprecedented capabilities and value for network engineers. These advancements are not just theoretical; they are practical solutions that are already being implemented in various aspects of network maintenance.
Quantum Computing: The Next Frontier
Quantum computing represents the next layer of computational capabilities that could revolutionize the intelligence, AI, and by extension, predictive network maintenance. With its ability to process vast amounts of data at speeds unimaginable with current technologies, quantum computing could make predictive analytics even more accurate and timely.
Ethical AI: A Growing Concern
As AI technologies become more integrated into network maintenance, ethical considerations around data privacy and security will continue to be a high-risk area. Network engineers and tech companies must be vigilant in ensuring that AI tools are developed and deployed responsibly.
Skill Evolution for Network Engineers
The future will require network engineers to adapt and evolve their skill sets. Understanding AI algorithms, data sources, and machine learning models will be as crucial as understanding networking protocols. Continuous learning, development, and adaptation will be key factors in staying relevant in this rapidly changing field.
Conclusion
The future of predictive network maintenance in the tech industry is bright, thanks to the continuous advancements in AI technologies. From machine learning to quantum computing, these technologies promise to bring about a paradigm shift from reactive to proactive maintenance practices. However, this future is not without its challenges, including ethical considerations and the need for skill evolution among network engineers.
Final Conclusion
In today's rapidly evolving tech landscape, the shift from reactive to proactive network maintenance is not just a trend but a necessity. Artificial Intelligence (AI) stands at the forefront of this transformation, offering many solutions that promise to redefine how network engineers approach maintenance tasks.
From AI-driven network monitoring tools that provide real-time insights to predictive analytics that forecast network behavior, the capabilities are vast and impactful. While adopting these advanced technologies comes with its own challenges, in other areas, such as data privacy concerns and ethical considerations, the benefits far outweigh the risks. Reduced maintenance costs, improved network performance, and enhanced security are just a few of the major ones.
Looking ahead, the future is filled with possibilities. Advancements in machine learning algorithms, the advent of quantum computing, and the growing focus on ethical AI are set to further elevate the role of AI in predictive network maintenance. For network engineers, this means an inevitable evolution of skills and practices and an exciting opportunity to be part of a transformative journey that could revolutionize the tech industry.
As we navigate this promising yet complex landscape, one thing is clear: AI is not just an auxiliary tool but a pivotal element in modernizing network maintenance strategies. Its potential to turn the tables from reactive to proactive approaches is not just a theoretical concept but a practical solution already making waves in the industry.
So, whether you're a network engineer, a tech enthusiast, or a business leader, the message is clear: Embrace AI, understand its capabilities and limitations, and involve yourself in a future where technology doesn't just support but leads the way in innovation and efficiency.