AI in Network Management: Transforming Troubleshooting and Predictive Analysis
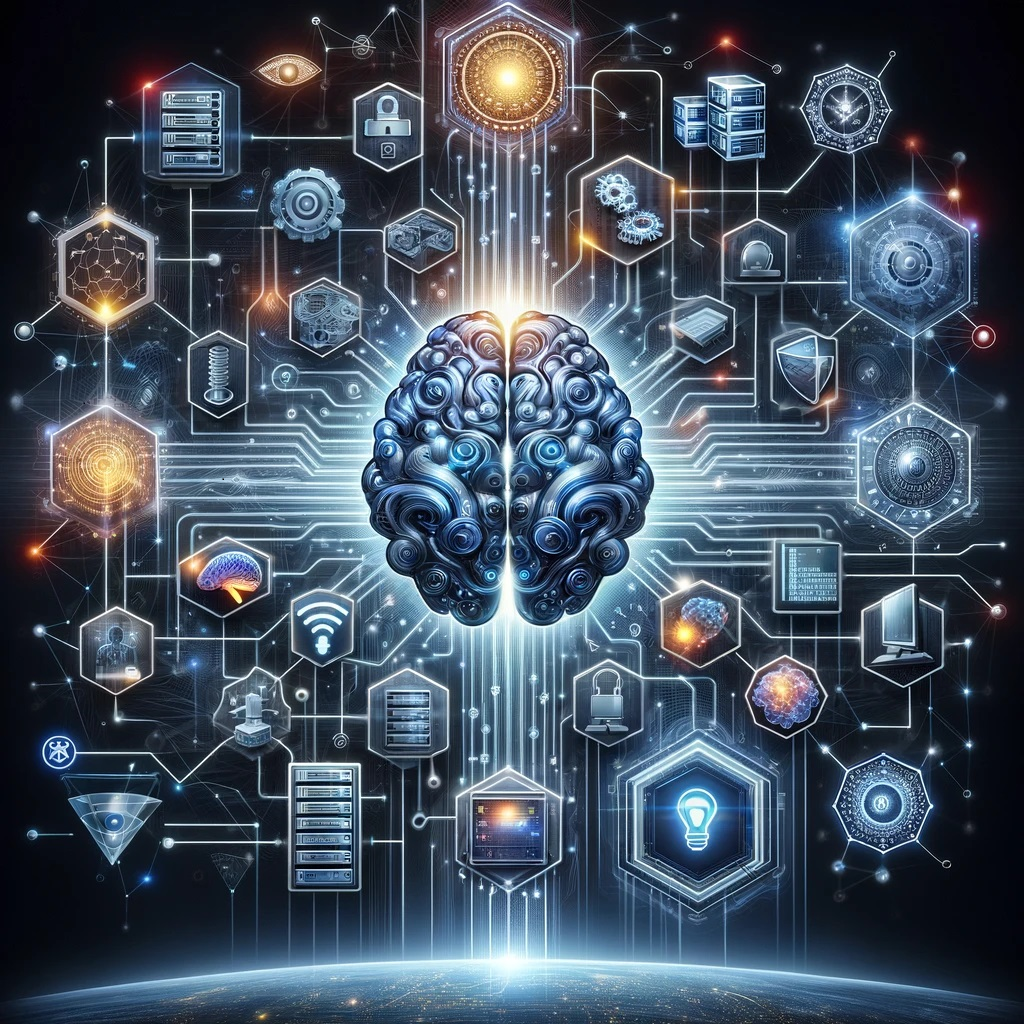
Introduction
Incorporating Artificial Intelligence (AI) into network management represents a major leap towards smarter digital infrastructure handling. Traditional network management methods often struggle to meet today’s complex digital demands. They typically react to problems after they’ve occurred, which can lead to expensive downtimes.
AI changes this dynamic entirely. It brings in a proactive approach, empowering network engineers to identify potential network issues before they cause major disruptions. This isn’t just a small step forward; it’s a complete transformation. AI does this by analyzing network data in real-time, spotting patterns and anomalies that might go unnoticed by human engineers.
This shift to AI-driven solutions means networks are not just stable; they're also more efficient and secure. It turns the network from a static setup into a dynamic, strategic asset. In a nutshell, for any organization aiming to excel in the digital landscape, AI in network management isn’t just nice to have—it’s essential.
This blog post will examine how AI is reshaping network management, focusing on its role in troubleshooting and predictive maintenance and what this means for the digital world’s future.
The Need for AI in Network Management
In the realm of network management, traditional systems have long been the backbone of IT infrastructure, ensuring connectivity and stability across various digital landscapes. However, as technology has advanced and network environments have become increasingly complex, these traditional systems face many challenges that compromise their effectiveness and efficiency.
- Scalability Issues
One of the most pressing challenges for traditional network management systems is scalability. As organizations grow, so too do their network demands. Traditional systems, often rigid and static in their design, struggle to adapt to this growth, leading to performance bottlenecks and increased downtime. The inability to scale effectively can hinder an organization's ability to innovate and respond to market demands. - Limited Visibility
Traditional network management often provides limited visibility into the network's overall health and performance. This lack of transparency can obscure potential issues, making identifying and addressing problems before they escalate difficult. IT teams are left in the dark without comprehensive visibility, reacting to issues rather than proactively managing them. - Inefficient Troubleshooting
Troubleshooting in traditional network management systems can be a time-consuming and resource-intensive process. Relying on manual diagnostics and interventions, IT teams often face lengthy resolution times, during which network performance and user experience can significantly deteriorate. This inefficiency impacts operational productivity and adds to the overall cost of network management. - Reactive Approach
Perhaps the most significant limitation of traditional network management is its inherently reactive nature. These systems are designed to respond to issues as they occur rather than prevent them. This approach can lead to repeated outages and performance issues, affecting business continuity and eroding stakeholder trust. - Security Vulnerabilities
With the increasing sophistication of cyber threats, traditional network management systems often fall short in providing robust security measures. Their reactive security protocols struggle to keep pace with evolving threats, leaving networks vulnerable to attacks that can compromise data integrity and privacy. - Inadequate Data Analysis
Finally, traditional systems cannot typically analyze vast amounts of network data effectively. In an era where data-driven decisions are paramount, this limitation prevents organizations from harnessing their network data to gain insights, optimize performance, and predict future trends.
In summary, while traditional network management systems have served as the foundation for network operations, their limitations in scalability, visibility, troubleshooting efficiency, proactive management, security, and data analysis underscore the need for a more advanced approach. Artificial Intelligence (AI) in network management emerges as a pivotal solution to these challenges, offering the potential to transform network operations from a reactive, manual process to a proactive, automated, and data-driven practice.
How AI Enhances Network Troubleshooting
Artificial Intelligence (AI) is revolutionizing network troubleshooting, shifting the network engineers' paradigm from manual diagnostics and reactive measures to proactive and predictive maintenance. By integrating AI into network management, organizations can significantly improve the efficiency and effectiveness of their troubleshooting processes. Here's how AI enhances network troubleshooting, illustrated with examples:
1. Automated Issue Detection
AI algorithms are adept at continuously monitoring network traffic and performance metrics to identify anomalies that could indicate underlying issues. Unlike traditional systems that rely on threshold-based alerts, AI can detect subtle deviations from normal network usage patterns beforehand, often identifying problems before they impact network performance. For instance, an AI troubleshooting network system can automatically detect an unusual spike in latency or a sudden drop in bandwidth, triggering an investigation even before users notice any degradation in service.
2. Predictive Analysis for Preventive Maintenance
AI's predictive analytics capabilities allow for identifying potential future issues based on historical data and trends. By analyzing past incidents and their precursors, AI systems can forecast likely future failures, enabling IT teams to address them before they occur. A concrete example of this is predicting hardware failures in routers or switches. AI can analyze error logs, performance metrics, and other indicators to predict when a piece of hardware will likely fail, allowing for its replacement during planned maintenance windows rather than after a disruptive failure.
3. Root Cause Analysis
AI enhances network diagnostics by rapidly pinpointing the root cause of issues, which can be incredibly time-consuming when done manually. Through analyzing vast amounts of data and applying machine learning models, AI can correlate disparate events and identify underlying problems that are not immediately obvious. For example, an AI system might analyze patterns in network traffic to identify a misconfigured firewall rule causing intermittent connectivity issues, which could take days for human analysts to discover.
4. Self-Healing Networks
Some AI network management systems have self-healing capabilities, meaning they can automatically take corrective actions to resolve identified issues without human intervention. This could include rerouting traffic to avoid a faulty network segment, adjusting QoS settings to mitigate congestion, or restarting a service that's become unresponsive. This speeds up resolution times and reduces the operational burden on IT staff.
5. Enhanced Security Diagnostics
AI also plays a crucial role in enhancing network security diagnostics. By analyzing patterns and detecting anomalies, AI systems can identify potential security breaches or vulnerabilities that traditional security measures might not catch. For instance, an AI system might detect patterns of data exfiltration or a series of failed login attempts from an unusual location, triggering alerts and automated responses to mitigate the threat.
Example: AI-Powered Network Optimization
Consider a scenario where an organization's network experiences frequent, unexplained slowdowns that impact user productivity. Traditional troubleshooting methods might involve manually checking network configurations, hardware status, and logs to identify the issue, which could take hours or days. With an AI troubleshooting network solution in place, the system could automatically analyze traffic patterns, identify the bottleneck causing the slowdowns, and suggest or implement configuration changes to resolve the issue, all within minutes.
In summary, integrating AI into network troubleshooting—through automated issue detection, predictive analysis, root cause analysis, self-healing capabilities, and enhanced security diagnostics—represents a significant leap forward in network management. AI improves the efficiency and effectiveness of troubleshooting efforts and enables a more proactive and less disruptive approach to network maintenance, ensuring higher availability and better performance of critical network infrastructure.
Predictive Analysis in Network Management
Predictive Network Analysis, powered by Artificial Intelligence (AI), is reshaping how network management is approached, moving beyond traditional reactive measures to a more proactive and strategic framework to optimize networks throughout. This advanced analysis method leverages AI to predict potential network failures and performance issues before they occur, allowing for preemptive action to avoid disruptions and optimize network performance.
Understanding Predictive Analysis with AI
At its core, predictive analysis involves using data, statistical algorithms, and machine learning techniques to identify the likelihood of future outcomes based on historical data. In the context of network management, this means analyzing patterns and trends from network data to foresee potential issues. AI enhances this process by automating data analysis at scale and with greater accuracy, enabling network managers and systems to learn from past behaviors and predict future states.
Benefits of Predictive Network Analysis
- Proactive Problem Resolution: Perhaps the most significant advantage of predictive network analysis is its ability to identify and address problems before they impact the network. By predicting potential points of failure, IT and security teams can implement solutions in advance, drastically reducing downtime and improving network reliability.
- Optimized Network Performance: AI-driven predictive analytics continuously monitors network performance, identifying trends that may indicate future performance bottlenecks. This allows for adjustments to network bandwidth to be made in advance, ensuring optimal network performance and a better user experience.
- Enhanced Security Measures: Predictive analysis extends into network security, where AI models can detect patterns indicative of security threats. Organizations can fortify their defenses and prevent data breaches by predicting potential security breaches before they happen.
- Cost Savings: Predictive network analysis can save significant costs by reducing downtime and improving network efficiency. Proactive maintenance helps avoid expensive emergency repairs and optimizes network resources, leading to more efficient operations and improved performance.
- Data-Driven Decision Making: With network predictive analytics, decisions are based on comprehensive data analysis rather than intuition or reactive measures. This data-driven approach ensures that network policies and strategies align with actual network behavior and requirements.
Implementing Predictive Network Analysis
Implementing predictive analysis in network management involves integrating AI and machine learning(ML) models to process and analyze large volumes of network data. These models are trained on historical network performance data, learning to identify patterns and anomalies that precede network issues. Once implemented, these systems continuously monitor network activity, providing real-time insights and predictions that guide proactive network management.
In conclusion, predictive network analysis, powered by AI, is transforming network management from a traditionally reactive discipline into a proactive, strategic function. By leveraging the predictive capabilities of AI, organizations can enhance network reliability, optimize performance, secure their infrastructure, and make informed, data-driven decisions. This strategic approach improves operational efficiency and supports the evolving demands of modern digital ecosystems.
AI's Role in Optimizing Network Performance
Integrating Artificial Intelligence (AI) into network management has significantly advanced the capabilities for optimizing network performance. This transformative technology leverages sophisticated algorithms and machine learning to enhance every aspect of network operations, from increasing efficiency and reducing downtime to ensuring seamless user experiences. Below, we explore how AI contributes to network performance optimization and highlight some real-world applications.
1. Real-Time Monitoring and Analysis
AI continuously improves network performance by scrutinizing network metrics and detecting anomalies that hint at issues before they escalate. This streamlines network operations and ensures optimal performance through intelligent, data-driven insights.
2. Predictive Maintenance
Leveraging historical data, AI predicts future failures, allowing for preemptive action. This reduces downtime and maintains network efficiency, exemplifying AI's predictive power in sustaining network health.
3. Traffic Optimization
AI dynamically manages network and traffic flows, prioritizing essential data and adjusting bandwidth allocation. This capability ensures smoother data flow and enhances user experiences, particularly in high-demand scenarios.
4. Security Enhancements
AI bolsters network security by swiftly identifying and neutralizing threats. This proactive defense mechanism keeps networks safe and operational, highlighting AI's critical role in cybersecurity.
Real-World Applications
- Telecommunications: Major telecom companies use AI to monitor network performance, predict hardware failures, and dynamically allocate bandwidth based on demand, significantly improving service quality and customer satisfaction.
- Cloud Services: Cloud service providers leverage AI for load balancing and to predict and mitigate service outages, ensuring high availability and performance for hosted applications and services.
- Finance Sector: Financial institutions employ AI to secure their networks against fraud and cyber-attacks, protecting critical financial data and ensuring smooth operations.
In conclusion, AI's role in optimizing network performance is multifaceted, offering significant improvements over traditional network management approaches. Through real-time monitoring, predictive maintenance, traffic optimization, and security enhancements, AI ensures that networks are more reliable, efficient, and secure. As technology continues to evolve, the adoption of AI in network management is set to become standard practice, driven by its proven ability to enhance network performance and support the growing demands of the digital world.
Empowering Network Management with SliceUp's AI Solutions
At the forefront of integrating Artificial Intelligence (AI) into network management, SliceUp is revolutionizing how networks operate, troubleshoot, and anticipate future challenges. By harnessing the power of AI, SliceUp offers an innovative suite of solutions designed to optimize network performance, enhance security, and ensure unparalleled reliability. Our platform goes beyond traditional network management methods, employing predictive analytics and automated troubleshooting to address issues before they impact network functionality proactively.
SliceUp's AI-driven approach enables real-time monitoring and analysis of network infrastructure, identifying patterns and anomalies that could signify potential problems. This level of insight allows for predictive maintenance, ensuring that networks are reactive, resilient, and prepared for future demands. Furthermore, SliceUp's solutions dynamically optimize network traffic and manage bandwidth allocation, significantly improving data flow and reducing congestion. In an era where cybersecurity threats are ever-present, our platform enhances network defenses, using AI to detect and mitigate threats swiftly, safeguarding critical data and infrastructure.
By partnering with SliceUp, organizations can transition from a reactive network management model to a proactive and predictive framework. This shift reduces downtime and operational costs and elevates network performance to meet the demands of modern digital ecosystems. Whether it's telecommunications, cloud services, or financial operations, SliceUp's cutting-edge technology empowers businesses to leverage their network as a strategic asset, driving innovation and ensuring a competitive edge in the digital landscape. With SliceUp, network management's future is redefined through the lens of AI and predictive analysis.
Conclusion
Artificial Intelligence (AI) is revolutionizing network management by transitioning from traditional, reactive approaches to proactive and predictive methodologies. This shift enables real-time network monitoring, predictive maintenance to address potential issues preemptively, and dynamic traffic optimization to ensure efficient data flow and enhanced security measures against evolving cyber threats. These AI-driven capabilities significantly improve network reliability, performance, and security.
The importance of AI in network management cannot be overstated. By leveraging AI's power, organizations can anticipate and solve network issues before they escalate, ensuring uninterrupted service and optimal performance. This predictive analysis and automated troubleshooting save time and resources and provide a competitive edge in today's fast-paced digital environment.
In conclusion, AI's role in network management marks a pivotal development towards more intelligent, resilient, and efficient network infrastructures. As we look to the future, the integration of AI in network management will undoubtedly continue to grow, driving innovation and transforming the digital landscape. This evolution underscores the critical need for organizations to embrace AI technologies to enhance their network operations and prepare for the demands of tomorrow's digital demands.